Pooling medical data could save countless lives. Artificial intelligence (AI) has the potential to transform our understanding of biology, optimize treatment decisions, and outperform humans in diagnosing illnesses like cancer. However, in order to work, AI algorithms need to be trained on large datasets.
Unfortunately, valuable health data is often dispersed over multiple data silos, each controlled by a different entity.
Examples may include GP surgeries, hospitals, labs, health insurers, pharma companies, and even the smart health gadgets many of us wear.
While these entities can apply their own AI models to their generated data, their models could benefit significantly from the data held in other silos.
However, for practical, business, IP or legal reasons, sharing the data (such as with federated data approaches) or the models (such as with federated learning) is impossible or highly controversial.
The NHS recently proved this point when it tried to create the world's most valuable health dataset.
The plan was to centralize the GP records of more than 55 million patients. Though officials declared the NHS would pseudonymize the data, it remains possible to identify patients. Once the privacy ramifications became clear, public outrage forced the NHS to pause its plans.
This is not the first initiative to collect healthcare data on a massive scale, and we suspect it will not be the last.
One approach to preserve user privacy is called federated machine learning. Companies and researchers, however, often reject this approach because it relies on sharing valuable AI models.
Apple's virtual assistant Siri is the most famous example of how federated machine learning works. First, each user trains their version of Siri on their device. Then, apple collects users' models and uses this information to improve the master model. This approach works for Apple because only one model is involved, but it becomes complicated when multiple models from multiple parties would be involved, since they would need to share their intellectual property.
This issue has limited medical progress.
Imec is pioneering a new approach called ‘privacy-preserving amalgamated machine learning’ (PAML). PAML allows each participating entity to build their own model using only locally available data whilst indirectly incorporating information from other data silos in a way that does not compromise privacy or harm commercial interests.
We consider PAML to be a significant technical breakthrough and hope it will soon be widely adopted by the medical community to ethically and sustainably advance science.
Read more:
- Our publication on how AI advances can enable medical research without sharing personal data on World Economic Forum
- Our article on how data and life sciences could fall in love
- VentureBeat's article on how amalgamated learning could scale medical AI
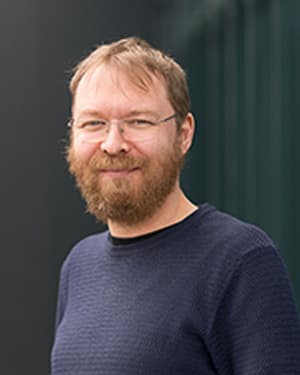
At imec Roel Wuyts leads the ExaScience Life Lab, a lab focused on providing software solutions for data-intensive high-performance computing problems, primarily in (but not limited to) the life sciences domain. He is also a part-time professor in the Distrinet group at the KULeuven. His academic achievements include published papers in IEEE Software or the Journal of Systems and Software, TOPLAS, ECOOP, OOPSLA or AOSD. He still has a special place in his heart for dynamic programming languages and was the organizer of the first Dynamic Language Symposium (DLS), co-located with OOPSLA'05.
More about these topics:
Published on:
29 October 2021